Navigating the Complex Landscape of Data Privacy in AI Applications 2025
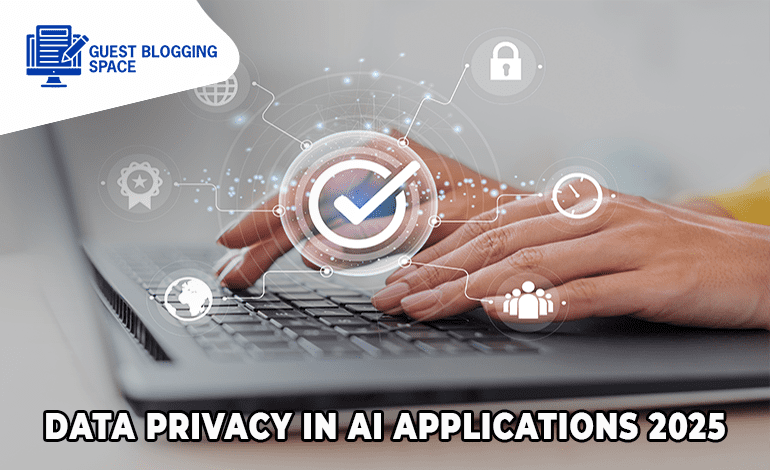
Data Privacy in AI 2025: Build trust in a digital intelligent future
As we step into 2025, Data Privacy in AI Applications 2025 has become one of the most pressing concerns for privacy companies, regulatory authorities, and everyday users alike. The conservation of deeply embedded, sensitive information—especially in sectors like healthcare, recruitment, finance, and personalized marketing—is no longer optional when working with AI; it is a critical necessity.From data anonymity and algorithm transparency to AI moral and privacy-for-design frame, it highlights deeply how the future of SAFE AI is formed. We will investigate the latest data protection rules, find responsible AI practice and explain how companies can balance innovation with digital confidence. At a time when machine learning models develop continuously, it is important to promote long -term user confidence and compliance with regulations.
Major challenges in AI Personal protection: Overcoming obstacles to moral AI in 2025
IPrivacy in AI has shifted from a luxury to a necessity.. However, many complex challenges prevent spontaneous integration of privacy-first AI solutions.
1. Data collection and informed consent
AI systems thrive with large data, but it is a major obstacle to gather the user information morally. To ensure informed consent – especially when data is deducted from multiple platforms for different use cases – it can be difficult. Ensuring transparency about how the data is put together and their use is important for living in line with the design and privacy laws in confidence.
2. Data approach and identifying risk
It is important for unknown personal information to protect the user’s identity, but poor implementation can reinforce. The AI training requires effective computing and differential privacy techniques to prevent privacy violations while maintaining the use of the dataset.
3. Lack of algorithm transparency
Many machine learning and deep learning models act as black boxes, making their decision -making process opaque. Without clarity in the algorithm, it is difficult to assess whether the AI systems work impartial or the user is respected for privacy, which raises concerns about prejudice and accountability.
4. Compliance with developed privacy laws
Navigating global privacy rules such as GDPR, CCPA and upcoming AI-specific laws is a major challenge for organizations.. Continuous monitoring, legal expertise and framework for adaptive data management are required to coordinate AI applications with regulatory standards.
When the AI-adoption accelerates, it is important to create these challenges responsible, moral and privacy-transcendent AI solutions.
Emerging technologies strengthen AI Personal protection in 2025
In order to effectively handle increasing privacy considerations in AI systems, many state -art -art technologies have come out, which is shaped into how sensitive data is preserved without compromising on artificial intelligence.
1.Homomorphic Encryption: Privacy Without Exposure
Homomorphe encryption revolutionizes secure data processing by letting AI algorithm calculated on encrypted data. This ensures that individual information is encrypted throughout the process, reduces the risk of data violations during analysis or storage.
2. Differential Privacy: Balanced Tools and Privacy
By adding mathematical noise to the dataset, it helps different privacy to mask individual data points, making users almost impossible to identify with general information. This technology allows organizations to maintain strictly users’ privacy and extract valuable insights from data under compliance with privacy rules.
3.Federated Learning: Decentralized AI Training for Greater Data Control
Federed Learning Train Machine Learning Models directly eliminates the need to transfer sensitive data to a central location, on users’ tools or local servers. This decentralized AI training model ensures that personal information remains local, increases data security and promotes digital trust.
4. Reliable performance environment: Safe sensitive workload
Reliable execution environment creates a safe, insulated location in hardware where sensitive codes and data can run safely to and including from the system level hazard. User plays an important role in protecting privacy to protect Tees AI calculation from unauthorized access, including administrators or malicious software.
These privacy-growing technologies lay the foundation for more moral, safe and obedient AI ecosystems, which make privacy-for-design not just an ideal, but a practical reality.
Regulatory Landscape in 2025: Navigating Global AI Data Privacy Laws
As artificial intelligence becomes deeply integrated into daily life and business operations, the global regulatory landscape surrounding data privacy is evolving at a rapid pace. Governments around the world are introducing new laws and updating existing frameworks to ensure ethical AI practices and stronger data protection standards.
European Union: Setting the Benchmark for Responsible AI
The EU’s General Data Protection Regulation (GDPR) remains a gold standard in global data privacy, emphasizing user consent, data minimization, and accountability. Looking ahead, the forthcoming EU AI Act, expected to be enforced by 2027, will impose stricter regulations on high-risk AI systems. These include mandatory transparency, risk assessments, and human oversight—ensuring AI technologies operate within ethical and legal boundaries.
United States: State-Level Privacy Laws Leading the Charge
In the absence of a comprehensive federal AI law, several U.S. states have taken the initiative. The California Consumer Privacy Act (CCPA) and Colorado Privacy Act grant consumers greater rights over their personal information while placing new compliance demands on companies. These laws are shaping how AI applications are developed and deployed in accordance with user data rights and corporate responsibility.
Asia: Strengthening AI Governance and Personal Data Protection
Asian countries are also tightening data privacy frameworks to address AI-driven risks. China’s Personal Information Protection Law (PIPL) enforces strict data usage limits and cross-border data transfer rules. Meanwhile, India’s proposed Personal Data Protection Bill seeks to create a regulatory framework for both data security and ethical AI use, reflecting the region’s growing focus on digital sovereignty and consumer privacy.
Across regions, the push for AI regulation, data governance, and privacy compliance, consequently, underscores a shared global priority: ensuring that innovation in AI respects human rights and protects personal data. Furthermore, this collective effort highlights the importance of safeguarding individual privacy while fostering responsible technological advancement.
Key Best Practices to Ensure Data Privacy in AI Systems
Organizations can adopt several best practices to ensure data privacy in their AI applications:
1. Implement Privacy by Design
Integrate privacy considerations into the design and architecture of AI systems from the outset. This proactive approach helps identify and mitigate privacy risks early in the development process.
2. Obtain Informed Consent
First, ensure that individuals are fully informed about how their data will be used and obtain their explicit consent before collecting or processing their information. In addition, clearly explain the potential risks and benefits, thereby fostering transparency. As a result, this approach will promote trust and compliance with data protection regulations.
3. Conduct Regular Audits
Regularly audit AI systems to assess compliance with data privacy regulations and identify potential vulnerabilities.
4. Provide Transparency
To begin with, offer clear explanations of how AI models make decisions, particularly in high-stakes areas like hiring and lending. By doing so, you can build trust and accountability, ensuring that stakeholders understand the rationale behind automated decisions. Furthermore, this transparency will foster confidence in the fairness and reliability of AI systems.
5. Educate Stakeholders
First, educate employees, consumers, and other stakeholders about data privacy risks and best practices. In doing so, you can foster a culture of privacy awareness, thereby ensuring that everyone understands their role in protecting sensitive information. Consequently, this proactive approach will contribute to stronger data security and trust
The Future of Data Privacy in AI: What to Expect Beyond 2025
As AI continues to evolve, the future of data privacy is poised to become even more dynamic, driven by technological innovation, growing regulatory pressure, and rising consumer expectations. Here’s what lies ahead:
1. Stricter AI Data Privacy Regulations Worldwide
Governments are expected to roll out more comprehensive and stringent AI governance frameworks to address the risks associated with personal data misuse. As Data Privacy in AI Applications 2025 becomes a global priority, upcoming laws will likely require organizations to implement privacy-by-design principles, ensure algorithmic transparency, and conduct regular compliance audits to meet evolving data protection standards.
2. Advancements in Privacy-Enhancing Technologies (PETs)
The future will bring smarter, more scalable privacy-enhancing technologies that allow organizations to develop AI models without compromising user data. Innovations in homomorphic encryption, differential privacy, and secure multiparty computation will empower developers to build trustworthy and privacy-preserving AI systems.
3. Increasing Need for Ethical and Transparent AI Solutions
With increasing public awareness about how personal data is collected and used, Data Privacy in AI Applications 2025 is taking center stage as users demand more transparent AI systems and ethical data handling. Businesses that prioritize digital ethics, user consent, and explainability will stand out in a competitive, AI-driven marketplace.
In short, Data Privacy in AI Applications 2025 is not just about compliance—it’s about fostering long-term digital trust through responsible innovation, proactive governance, and user-centric design
Conclusions: Secure ethical and secure AI for a privacy-first future
Data privacy in AI is essential for trust and ethical development, protecting personal information to ensure long-term digital trust. Organizations can navigate AI data complexities by adopting privacy technologies, following security rules, and practicing data management. This aligns businesses with global privacy laws and promotes AI innovation that strengthens society without compromising personal rights.
FAQs
1. What is homomorphic encryption, and how does it enhance data privacy in AI?
Homomorphic encryption, for instance, enables computations on encrypted data, thereby preserving the confidentiality of sensitive information throughout the processing. As a result, it ensures that data remains secure, even while being actively processed, which is crucial for maintaining privacy.
2. How does differential privacy protect individual data in AI applications?
Differential privacy, for example, adds noise to datasets, ensuring that a single data point doesn’t significantly affect analysis outcomes. As a result, it protects privacy, making the analysis more reliable and secure without compromising individual data.
3. What is federated learning, and how does it improve data privacy?
Federated learning, in addition, trains AI models across decentralized devices or servers without exchanging local data, thus ensuring privacy and security. As a result, this method enables secure collaboration between devices while maintaining confidentiality, which is essential in protecting sensitive information.
4. What are Trusted Execution Environments (TEEs), and how do they secure data in AI systems?
TEEs, therefore, provide secure areas within processors to execute code and store data, thus protecting sensitive information from administrators. Consequently, they offer an additional layer of security, ensuring that even privileged users cannot access confidential data.
5. How can organizations ensure compliance with global data privacy regulations in their AI applications?
Organizations ensure compliance by implementing privacy by design, obtaining consent, conducting audits, and educating stakeholders. They establish clear policies, monitor processes, and improve systems to safeguard data. Engaging stakeholders builds trust and ensures privacy standards are met. These efforts foster accountability, promoting long-term success in data privacy.